'It doesn't add up!' Statistics and the fight for equality
19th December 2013
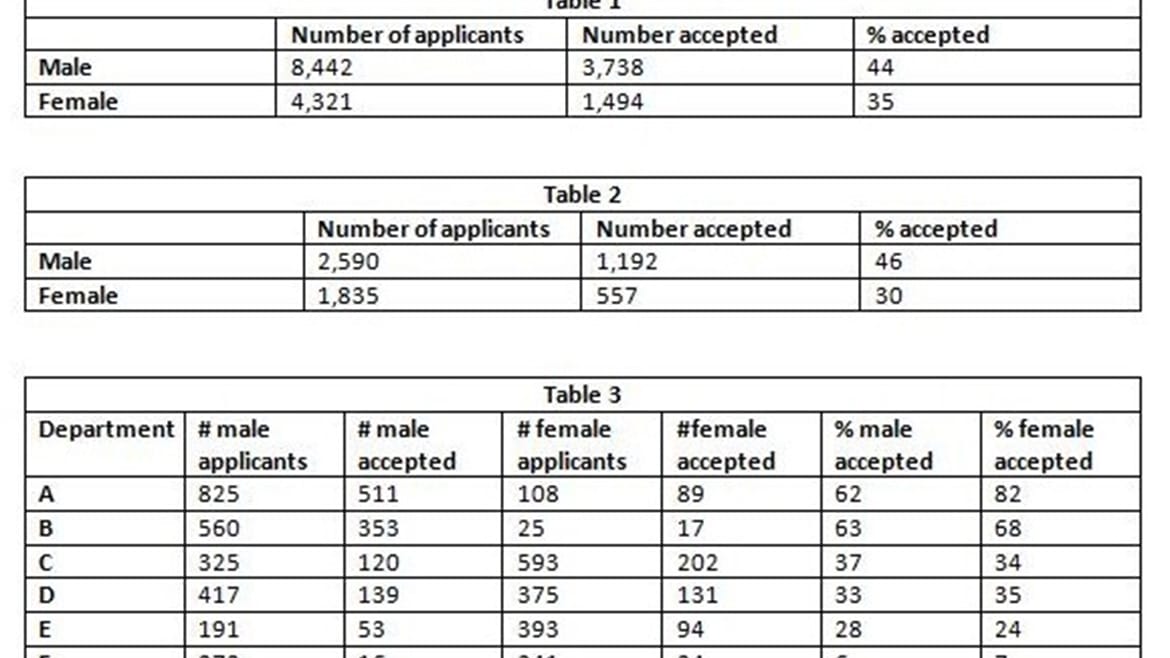
In the autumn of 1973, the University of California at Berkeley was sued for sexism against women. This wasn’t a new accusation, and in fact at the time universities often found themselves in the midst of similar controversies, but this time, it looked like there was irrefutable proof. The acceptance figures for applicants to graduate school were the ones recorded in Table 1 above.
This represents a very large difference between the rates of acceptance of male versus female applicants, a variation that is much beyond what could reasonably be expected to happen naturally if men and women were equally as likely to get in – something which can be verified by calculating what is called a p-value.
Once it was established that the gap between the acceptance rates was not a reasonable one, the university commissioned a team of three experts to conduct further research.
Admissions were handled independently by each department, and the experts identified the five departments which made up the largest variation in acceptance rates. Together the admission figures for these five were the ones you can find in Table 2 at the top.
The gap was even wider than for the university overall – but when the numbers were broken down per department, the evidence of sex discrimination all but vanished, as you can see in Table 3.
What we can see here is that none of the departments displayed a marked preference for male applicants, and in fact one accepted a far higher proportion of women than men.
This is an example of Simpson’s Paradox, a statistical paradox which actually shows up regularly in real life (and not just in exam questions). It is the name of a situation in which a trend that appears in different groups of data disappears, or even gets reversed, when these groups are combined. It happens when an important part of the information is lost when the data is aggregated.
Here, the information that gets lost is the fact that women applied in much smaller numbers than men to departments A and B, which were scientific departments and have a high acceptance rate (this does not mean that they are easier to get into, but rather that scientific subjects are more self-selecting!), and applied in much higher numbers than men to departments C, D, E, and F, arts subjects with a much lower acceptance rate.
This story is a good lesson in how aggregated data can be misunderstood or even manipulated into illustrating false arguments. There is no better way to make an informed decision than to consider the raw data instead of relying on sensational headlines and reports.
At Unifrog, we break our data down at course level so that students have access to objective and precise information and can reach their own conclusions. Want to know more about how Unifrog works? Please request a demo here (we’ll then set up a meeting with you).